Whether you outsource a deep learning solution to a vendor or bring that expertise in-house, here’s what marketers need to do to make deep learning work.
Technology continues to push the boundaries of what’s possible for marketers. While most marketing executives understand that software can drive growth, not everyone is familiar with the underlying technologies that power the latest tools.
That’s okay because your job is to put software to work, not put it together. But a basic understanding of how some of these tools work can allow you to make better decisions about where to invest your budget. With that in mind, let’s take a look at one technology that is typically accompanied by a lot of hype but little clarity: deep learning.
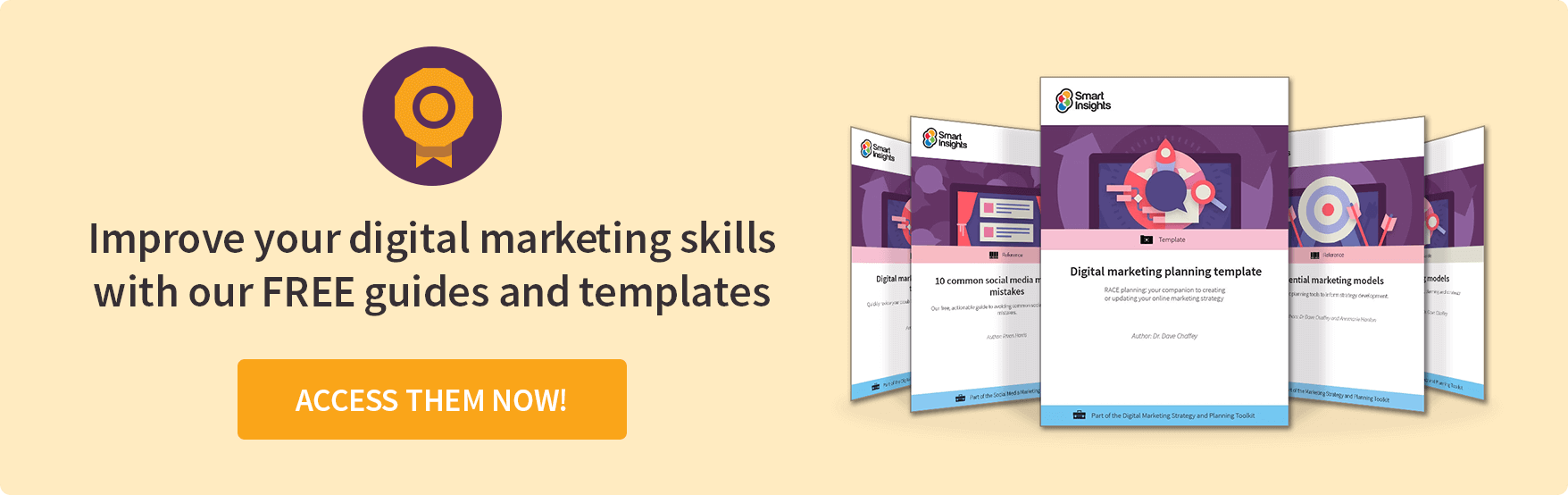
Making sense of deep learning
Modern marketing teams are concentrated on full-funnel engagements and want to track every interaction prospects have with their company through the website, content, events, and elsewhere. This data can potentially give them a holistic view of the factors and touchpoints that move prospects down the funnel to the sales team.
Unfortunately, today's marketing teams can suffer from something that only a decade ago would've been considered an enviable problem: too much information. This leads to a number of often contradicting models for gauging the return on investment of different strategies and a general lack of understanding about where and when to engage.
Enter deep learning. It's a particularly powerful form of machine learning based on neural nets (mathematic operators that simulate the structure of the human brain) and is extremely valuable when you have vast amounts of reasonably structured data. It can help marketers understand client interactions on a granular level and predict how those interactions will lead to particular outcomes.
Moreover, because deep learning can consider thousands of potential decision paths at once, the predictions are significantly more powerful than what can be done via traditional statistical methods. To get a better idea of how this is possible, let’s look at two use cases: lead prioritization and sales alignment.
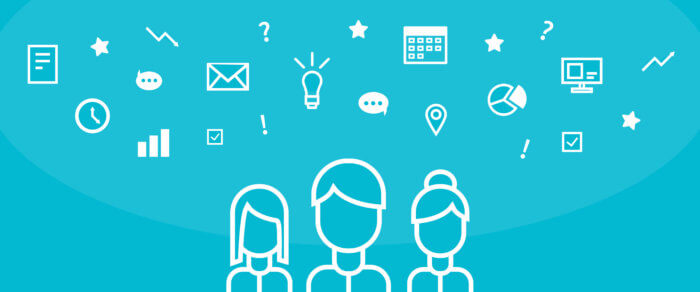
Lead prioritization
Traditional lead scoring revolves around two questions:
1. Is this company or person a good fit for our business?
2. What do lead actions tell us about interest?
It’s far from an exact science.
Consider two potential leads. Both are founders of startups, have C-level titles, and sell to similar industries. However, the non-founder executive teams have significantly different experiences and abilities.
Using traditional methods, you'd probably score these leads similarly, and your marketing would reach both. However, the ROI might be significantly different. With deep learning tools, a program can analyze different types of background information for each and interpret those factors in an interconnected way to tell you whether an individual matches your product rather than your persona.
Sales alignment
If you’re following an account-based marketing strategy, your focus is on a smaller number of top-tier accounts and the key stakeholders at each. Your goals aren’t necessarily the same as those of a traditional inbound marketer; they’re probably more tied to sales organization and supporting the processes that impact revenue.
Download our Business Resource – Digital marketing e-learning course
This interactive online course will help you or your team rapidly learn the fundamentals of successful digital marketing.
Access the Digital marketing e-learning course
Deep learning can tell ABM marketers which buyers associated with an account are most important to target, where other likely influencers sit in the account, and how likely an account is to convert. It can even help you align your resources around the success criteria that are most important to your organization.
Making deep learning work
Modern marketers are ahead of the curve in implementing artificial intelligence-powered tools, as just over half already rely on at least one to get customer insights or make workflows more efficient. Whether you outsource a deep learning solution to a vendor or bring that expertise in-house (if you already have a dedicated data science team), here’s what marketers need to do to make deep learning work:
1. Know what outcome you want to optimize for
With any type of deep learning, the most important thing is understanding what outcome you’re trying to optimize for and then building an effective training set that helps you get there. Maybe you want to understand what drives improvements in website click-through rates or what campaigns attract the highest-converting leads. With deep learning, there are countless interactions to investigate.
You should begin by clearly defining the problem you are trying to solve for and then identify your ideal outcome. What does success for this project look like? And what constitutes a failed outcome? Also, consider how you will use the output or information learned from machine learning. All of these steps will help you figure out what information you are seeking and how you plan to get there.
2. Get your data together
Deep learning is fueled by data, but the data that's fed into it (the training set) must be machine-readable. That means it must be translated into a consistent mathematical format to be evaluated. Historically, this process has required data science expertise.
To build an effective training set, you first need to determine what data you can use for the project and what data would be helpful that can be simulated to help solve for the problem. The most time-consuming part can be preparing the data to make it useful for machine learning. You must ensure the material is accurate to make it useful. And the more data you have, the more accurate the performance of machine learning will be.
Even if you’re not ready to invest in a deep learning solution today, get in the habit of collecting and storing customer data in an organized fashion. If you structure your data effectively now, you’ll have a head start when bringing in deep learning technology does make sense. Create connections between data silos at your company to mine special insights that can only be found by using multiple sources of data. Also, make sure you have the right to use the information. The General Data Protection Regulation in Europe restricts the use of some data, and several states are considering similar laws.
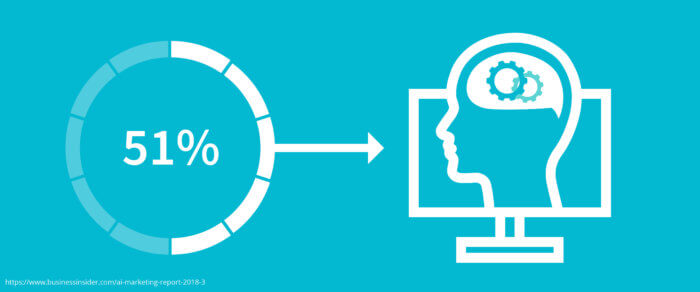
3. Decide how to implement your strategy
Whether you’re trying to connect with prospects and leads in real-time through chat, providing a follow-up communication like an email, or having sales reach out directly, you should have a plan to move them down the funnel. Deep learning can suggest which tactic is likely to be the most effective based on both explicit and implicit data. But it's ultimately on you as the marketing leader to interpret the predictions and implement the proper strategy.
One success story involving machine learning in marketing is cosmetics brand Sephora, which uses predictive modeling to customize its email marketing strategy. The company recommends products and extends offers and incentives to its most loyal customers based on previous purchases, which has led to a 70% increase in productivity. And it's all accomplished without a measurable increase in spend.
4. Accept the limitations, and monitor to minimize risk
Deep learning is incredibly powerful, but its inner workings are often opaque. The reason a particular conclusion was reached is often unknowable, even in principle. This limitation can be a tough pill for businesses to swallow because it limits accountability.
This limitation is one reason it's important to pay careful attention to the training process. Garbage data in means garbage predictions out. Even with good data, deep learning can amplify biases in the dataset. It's important to continually monitor and evaluate the predictions against the outcomes. That way, you can quickly address issues that arise as the result of ineffective or biased training.
Machine learning projects that use data sets that are constantly being updated produce the best results. So adopt a strategy that provides a continuous improvement of your data set. This also makes it harder for competitors to replicate your data set.
Marketers have a tough job, but deep learning can help companies better understand marketing data they have collected and use it to attract and engage clients. By following these steps to implement deep learning at your company, you can take advantage of this powerful technology.
Greg McBeth is the head of revenue at
Node.io, the first turnkey AI platform that enables businesses to leverage predictive artificial intelligence within their own products, customer databases, and external systems of record, all via a standard API. Greg also coaches companies and business professionals on how to improve performance. Connect with Greg on
LinkedIn and
Twitter.