How to make the most out of your company's data
Today’s corporate agendas revolve around the staggering amount of data available and the advanced analytics needed to gather, interpret and use such data. When it comes to Big Data and Advanced Analytics, things can get really tricky for a company not making the best of them.
A recent McKinsey report shows that businesses which are not able to leverage Big Data and which fail to achieve performing data management also witness an average of 14% in lost revenues per year. Surprisingly, oil companies, life sciences, and consumer goods make the top three industries where big data and advanced analytics are poorly managed, thus leading to financial losses.
As opposed to the McKinsey report, a study coming from MIT and Harvard Business School shows that companies focusing on big data management and advanced analysis witness an increase in revenue of up to 6% in comparison to companies not injecting big data into their financial and operational strategies.
But what are Big Data (BD) and Advanced Analytics (AA) when we refer to financial management and good practices? A broad description of BD defines it as being:
- Massive in volume and complexity, scaling up to multiple terabytes or even petabytes
- Unstructured and diverse
- Widely distributed both on the outside of the organization and inside the organization
- Fast moving and fast changing, needing perpetual sourcing and transmission.
In order to be able to source, understand and use BD, Advanced Analytics comes as a set of tools and techniques which are hard (if not impossible) to replicate with traditional analytics methods in size, accuracy, scale or scope.
Advanced Analytics go beyond simple regression or predictive models, using “new analytic methods” such as the study of social media buzz, data-mining, and others. The advantage of AA when it comes to predictions, however, is that (if used correctly) they can achieve higher levels of accuracy due to the larger data sample sizes.
AA strategies are based on tools and instruments helping CFOs improve the company’s performance on multiple levels. Let’s see today how CFOs implement AA and what good practices examples you can look into to adopt and adapt for your company.
1. Viewing the Budget in 360°
Using Big Data and Advanced Analytics can surely help a company stay ahead of the competition and get better at operations, financial management, sales forecasting, and consumer retention and so on. Optimizing data and analysis can help the CFO tackle with complex business rules, market shifts, and economic constraints. Thus, they become able to simultaneously evaluate them all and make better decisions.
However, before investing in AA frameworks, hardware, and data scientists, the CFO needs also to use BD to assess the company’s capability of implementing a BD and AA-centered approach.
While advanced analytics help the CFO translate business opportunities and constraints into mathematical formulas, charts and prediction models to create more accurate budgets and scenarios, the main issue is the company’s internal financial and managing architecture able to sustain the shift to the AA approach.
Such an endeavor can take years and needs a stellar coordination with all the departments in the company.
However, if the company is able to take the first steps towards such paradigm, CFOs need to pay attention to their environment and understand how to implement good practices.
2. Choosing the Right Data and Using it Creatively
All managers and CFOs are literally bombarded with data they sometimes deem unimportant. Other times they have access to the entire information they need, but don’t know where to look for it. The best practice in using advanced analytics on BD is finding the data in your environment and creatively choosing its sources.
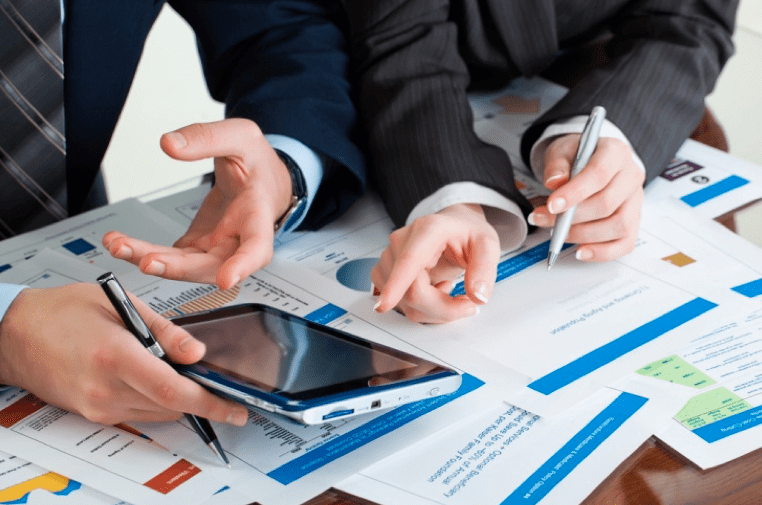
Given the fact that now we have massive amounts of unstructured information coming from new sources like social media and machine sensors, CFOs can become creative in picking the data sources and using them to improve working capital, resource allocation strategies, the management of costs, risks, human resources and revenues.
One example is of Sears Holding wanting to improve its promotions, personalized offers to customers and generate greater sales values.
But processing information coming from three sub-brands to tailor relevant and revenue-generating promotions in the traditional manner took around eight weeks, which was counterproductive for the company. Instead, Sears Holding switched to BD analysis using Hadoop cluster analysis (together with servers and software) to process the data and create revenue-generating, comprehensive and reliable product promotions in a week.
Other companies use social media, weather forecasts, electronic shipping records, customer surveys results, clients’ feedback and ratings.
3. Building Prediction Models
Prediction models are an instrument CFOs have been using traditionally to identify trends and patterns in market shifts, consumer behavior, and business performance. However, in the AA framework, prediction models should be constructed not starting with the data but with the identified business opportunities/weaknesses and with determining how the model can actually improve performance.
This shift in paradigm for CFOs can help them narrow the gap between strategic and operational decisions covering business levels that are not traditionally in the hands of the financial manager: supply chain optimization, customer retention, social media marketing planning, sales- and finance-linked forecasting, new product introduction profitability, customer satisfaction and so on.
For instance, instead of creating a complex product pricing strategy based on traditional data (historical sales data, price elasticity, market responses, competitors’ responses and so on), a company can develop a prediction model starting with the identification of factors that affect their sales. Then, they can determine what data and what model would best deliver the necessary insights for them to make price-related decisions.
What companies need to keep in mind when building models, however, is that they need to keep things simple, as models using 30-40 variables may exhaust its resources.
4. Changing the Company Culture
The CEO and the CFO may agree on using advanced analytics and complex models, but this doesn’t mean the employees will effectively use them in the front rows of operations. It is possible that while the CFO focuses on using AA to optimize the returns on advertising and marketing campaigns, the marketing team disregards the model, has little knowledge of how to correctly implement it or simply uses a different approach to decision making.
When using AA and tapping into big data, one of the best practices of a CFO is not only to implement new models but also make an impact on the company’s culture and practices changes. Granted, such approach is not a traditional one for a CFO, but in this day and age, one needs to think ahead and overpass the boundaries of the job description. Company culture change in relationship with AA can be achieved through a series of steps:
- Before implementing AA, the CFO needs to come up with an analytics tool that can be used by all departments at all levels. Speaking the same language and being on the same page (from human resources to online marketers) eases the company’s switch to using advanced analytics and prediction models.
- Complex software running through dozens of terabytes of data won’t surely make front row workers’ lives easier. One approach is embedding big data and complex analytics in simple and usable tools for the people working with such data minute by minute. As a CFO, it is your duty to optimize the company’s workforce performance and cut costs. Instead of worrying about the employee’s wasted time and resources to operate with complex data, it is cheaper and faster to use specialized IT companies to turn AA into simple, manageable tools.
- Invest in the employees’ big data literacy and management. All CFOs know it is cheaper and faster to train your employees than be faced with employee turnover just because the front row workers can’t handle the tools and techniques you have them operate with.
5. The Use of Crowdsourcing to Boost Forecasting Accuracy
CFOs typically have cross-views on the entire organization, keeping tight relationships with all departments, meaning they have a broad view of all employees’ capabilities and departments’ best resources.
Crowdsourcing is usually a sourcing model in which organizations use contributions from individuals to obtain ideas or services. However, when using Big Data we mentioned that it can be found both internally and externally.
A good practice when it comes to AA is internal crowdsourcing, meaning using the company’s employees’ skills and expertise to improve sales, fix prices, tailor promotions and generate revenue.
There is at least one excellent example of how a company can leverage on its internal data, employees’ wisdom, skills and expertise and prediction models to improve sales forecasts’ accuracy: Henkel. A practice used by Google and Microsoft, crowdsourcing in Henkel took the form of an employees’ vote on the next quarter sales for a batch of selected products.
The technique resulted in 22% improved sales forecast accuracy and the identification of experts within the company who perpetually registered high levels of forecast accuracy. Needless to say, identifying experts within a company and matching them to the right organizational position is profitable from all points of view.
Another example is that of Deutsche Telekom using employee’s evaluations of product concepts. Such an approach led to a very quick sales forecasting analysis which was reliable (a 84% correlation with a parallel consumer survey) and which was very low cost, allowing the company to save 90%.
Big Data and Advanced Analytics are still in their newborn years, offering endless possibilities for companies to use every single piece of information (inside and outside the organization) to better structure their business on all levels. In this framework, it is likely to witness major shifts in the roles and responsibilities of CFOs in the years to come.
Image sources: 1, 2, 3
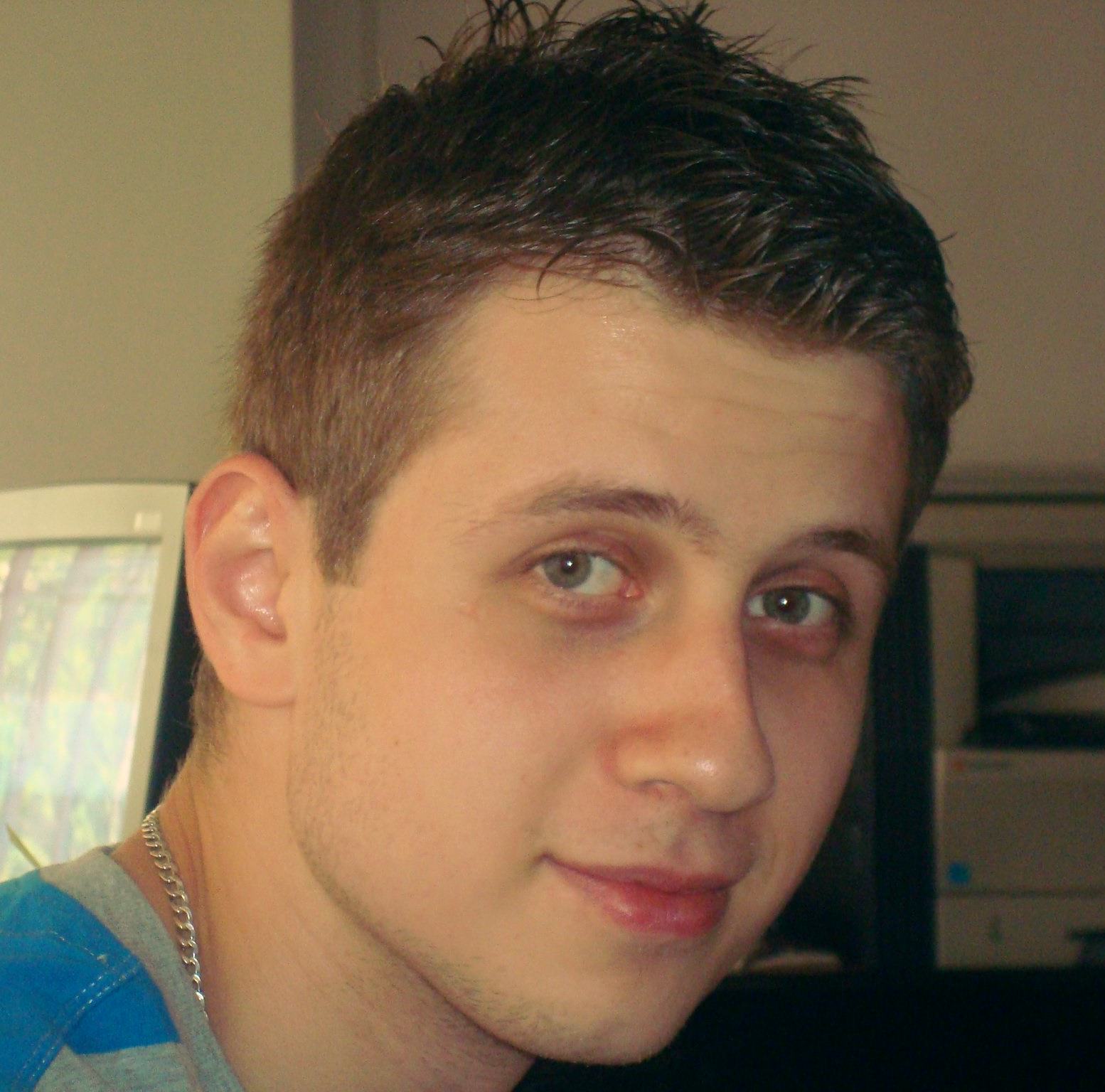
Thanks to Ethan Featherly for sharing his advice and opinions in this post. Ethan Featherly has a background in economics and trading. After he graduated from The University of Chicago he decided to become a financial analyst. Ethan is now a content manager at
Admiral Markets, where he is in charge of the educational section.