Using predictive analytics effectively will allow you to increase sales, but only if you have right data
Applying predictive analytics to marketing campaigns will enable businesses to increase sales and returns through visualising the campaign outcomes. Below is an introduction to Predictive Marketing and how you can begin to experiment with these ideas in your business.
Introduction
You may have heard of predictive marketing – it’s an increasingly popular topic. You may not, however, understand what it really means or how it could benefit your business.
In short: it involves using expected future events and expected future customer behaviours to create a marketing plan. It has become a lot more relevant to businesses as digital marketing campaigns have generated far more data which is analysed in far more detailed ways.
Intuitively, most marketers can see a clear value from understanding customers better and getting detailed numbers around a campaign’s expected returns before it has started.
However, the skills and processes required for predictive analytics projects can be difficult to develop and before you begin, you should know, if this really is for you.
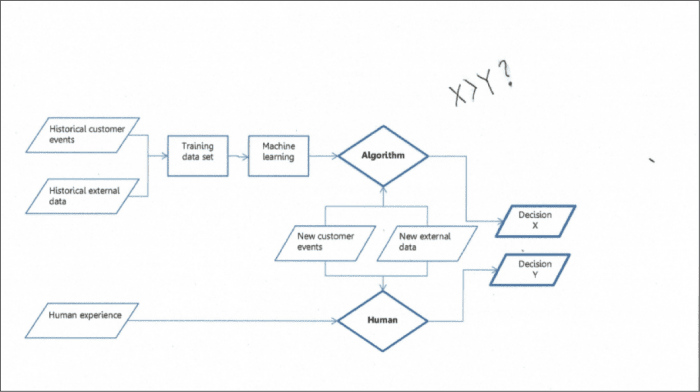
What is the potential?
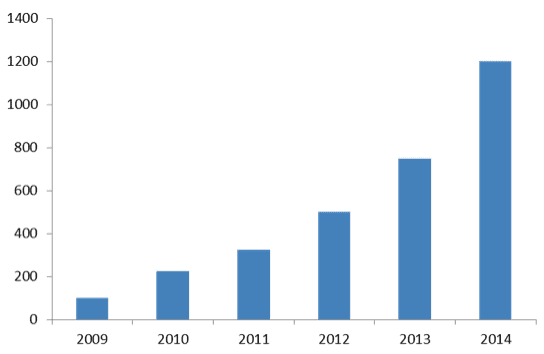
Figure 1 – Marketing automation platform sales ($m) - These have grown by approximately 1200% over the past 5 years. (source)
The potential to a business is based on the types of problems that can be solved. To begin with, it’s important to be clear that the problem is around propensity, i.e. the likelihood that an event will occur. This is in contrast to customer segmentation or recommendation problems – which require similar analytical techniques, but which involve describing current patterns (rather than predicting future outcomes).
As some examples that we have seen:
- A marketing consulting firm increased lead to sales conversions by shaping the correct products for each client. This data was gathered by monitoring client reactions through a lead generating website.
- A retail site reduced its bounce rate by 50% to a product category by predicting the landing page content that would be engaged with. The data was gathered through an analysis of the content referring visitors to the product category.
- A B2B technology business increased its email response rate by tailoring each to the predicted client problems.
- A professional services firm increased contact form conversions by changing format based on visitor location. The data was gathered through an analysis of a large set of high performing, international contact forms.
These can all be seen as generating value by increasing the value of each decision and action made. For example, by deciding which keywords to select in a PPC campaign or which colours to use on a product package. It relies on first organising marketing data correctly in order to quickly identify patterns and insights. The best organised data tends to enable the most successful digital marketing campaigns.
How this will vary between industries
As a rule of thumb, the more data available in the industry, the more potential exists for predictive marketing to provide new insights and benefits.
Although, what do we mean by data? In general the more transactions and interactions between customers and the businesses in an industry, the more potential data exists. The easier it is to record these interactions – for example, when they occur online – the more potential there is to harness this data for predictive purposes.
In addition, if the actions completed as a result of these predictions can be automated then that’s even better.
For example, a long-term online marketing campaign for a large online retailer like Amazon, has a large potential to be automated through predictive marketing due to the large stock of data available. Also, since the campaigns can all be controlled by machines, any decisions can be automatically implemented.
However, a new in-store event for a large supermarket may have less potential – even if you could collect the data (for example, through cameras and facial recognition of customers visiting the event), making a resultant decision and completing an action could only be done for the next day.
Since the optimisation cannot happen in real time, the value of the quick decision is reduced.
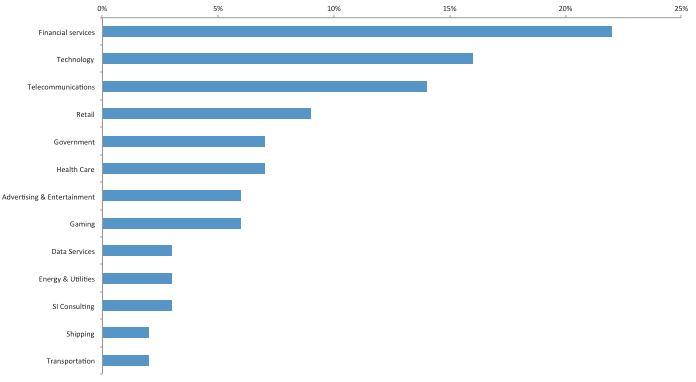
Figure 2 – Data Usage by Industry - Big data investment varies highly based on industry and the corresponding availability of data (source])
The power of learning
As industries exist for longer, the larger the available data sets become for those industries, which in turn creates a larger the scope for predictive marketing. In addition, as data is increasingly available around human interactions, due to increased connectivity and changes in regulations, the scope also increases.
For all industries we can therefore expect to scope for predictive marketing to increase as time goes on.
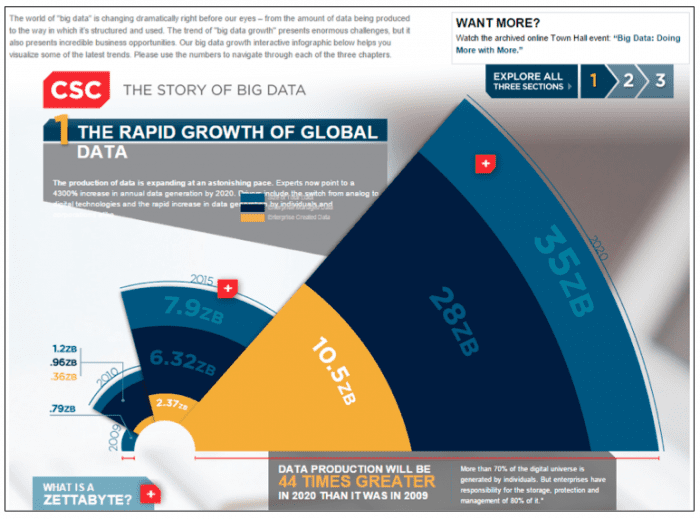
Figure 3 – CSC Infographic on the growth of global data – Growth in mineable data will enable more and more decisions to be made through predictive systems. (Source)
Will this affect a business’ profits?
Yes – in fact predictive marketing campaigns should not be undertaken unless there is a clear link between the decisions being made and the profitability of the business.
Whilst structuring a project, there should be a clear path between the decisions being made, the actions that will ensue and how this should increase the business’ profit. Predictive marketing, therefore is particularly powerful when looking at complex areas, where there are many possible ways forward.
Campaigns will improve – sometimes by many multiples – by reducing waste on actions that are not profitable, increasing investment on profitable actions and reducing the cost and time involved in making these decisions manually.
How do I know I will get meaningful answers from my investment?
It’s likely that a predictive marketing will generate value for your business if you are asking the right type of questions and you have enough data to complete a detailed analysis. In addition, this works best when you need to optimise for a particular decision and increased analysis can help you to close-in on the right answer.
The below is a short guide to some things that you should be thinking about.
- What choices do you wish to optimise for? Is there a clear problem that you are facing and that you have commissioned this analysis for? Predictive analysis is at its most useful when you are looking to select the best choice from a set of options.
- What is the list of possible options? Can you identify the universe of current options you have? For example, in a PPC campaign, keywords you can optimise for or in a PR campaign, the publications you can advertise in?
- How many case examples do you have for each? Do you have enough case examples involving each option to complete an analysis? It’s important that the data set contains enough examples of successes and failures which include possible actions that are under consideration.
- Which metrics are the better decisions designed to improve? Do you have a clear understanding of the metrics you are looking to improve (for example, CTR, sales conversions, etc) through this analysis and what represents success and failure with your decisions?
- Can this be tied back to profitability? Does the resultant improvement in project metrics result in increased profits for your business – is it therefore worth investing into?
It should also be noted that predictive analysis is more valuable when obtaining the answer has no unintended consequences. An example could be, if your action resulting from a prediction causes your customers to change their behaviour, therefore making your earlier analysis invalid.
Making a start: Regressions don’t have to be scary
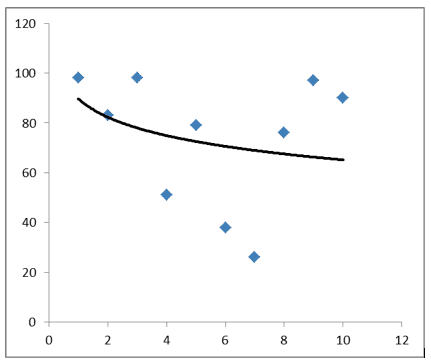
Figure 4 – Example of a simple trend line over a scatter graph generated by Microsoft Excel
In order to make a start in short time, you should create an initial project to investigate one question around a single trend.
This will involve building a regression model, although this doesn’t have to be too complicated. In fact some of Microsoft Excel’s built in tools can help you do this, with two basic lists of numbers (please see Microsoft's Excel guide here).
By starting an initial project you can begin to answer some simple questions and build up the basic skills and processes that you will need in the long term.
Please see our short guide below:
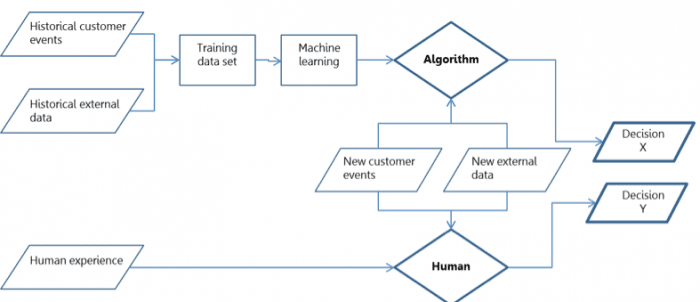
- Define and prioritise the questions - for example:
- Is there a link between our quarterly SEO spend and the number of organic visitors we generate?
- Which of our category pages is best at converting visitors to sales?
- What are the attributes of the individuals in our email data base, that signal that they are likely to respond to our messages?
- Complete a review of the problems and supporting data you have (see our list of 5 questions in the previous section).
- Find the right talent and divide your data
- Divide the historical data sets in to training, validating and testing sets, required for the model development.
- Find a mathematician and software engineer who can help you the build the basic model - this could be someone who has experience in applying predictive analysis to business or just someone who is good at using Microsoft Excel.
- Don’t be afraid to fail
It’s likely that your first short test will not surface any meaningful patterns. This should not be seen as a loss as it’s the first step in the researching of more complex hypotheses and insights that will generate value for you marketing campaigns.
Thanks to Sachit Patel for sharing their advice and opinions in this post.Sach is a Chief Data Officer at dcyfr. This is a new business which helps businesses increases lead generation and conversion rates through applying predictive analytics to online marketing campaigns. He can be found here.