How can Digital Marketers use SEO ranking factors correlations?
Love them or loathe them, correlation studies seem to be everywhere when discussing 'best practices' in SEO. So we have to ask - 'how can we use them' and 'should we trust them'?
Moz and Searchmetrics are the best known publishers of correlation studies who produce reports from sample sizes in the order of hundreds of thousands of webpages, with the purpose of identifying what’s important and what’s not in the world of search marketing.
So how do you use them to inform your online strategy - do you try to remove yourself from all human emotion and blindly go with these numbers or do you ignore them and trust your gut instincts? Or is there a middle ground? You can see the challenge from this recent Searchmetrics SEO Ranking factors infographic.
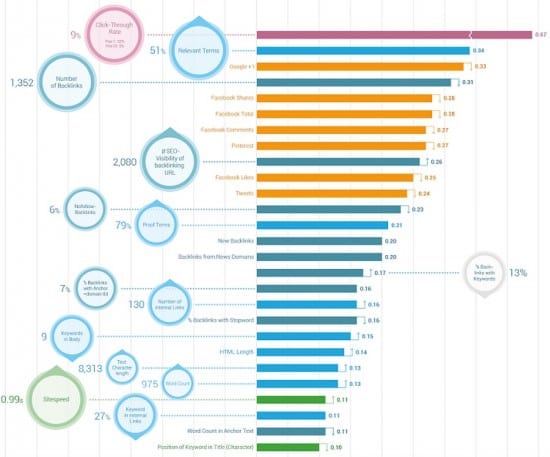
This infographic highlights some of the dangers of relying on ranking factors correlation compilations without wider knowledge and your own tests. For one, correlation doesn't equal causation. Sharing on social networks have a strong correlation, yet this may simply be because of the quality of content which increases dwell time on the post - a factor not shown here. Likewise the occurrence of keywords within the title tag of a post or anchor text linking to a post remain important, yet they aren't clearly shown here. And you will likely fall foul to the Penguin filter if you generate too many links with the same anchor text linking to one page. Subtleties you just can't see from a chart.
Don’t see them as a means to trick the search engines into liking you
Long gone are the days where marketers could realistically expect to understand the interplay between all the variables explicitly considered in a search engine’s algorithm - even if one had the resources to achieve the modern day equivalent, the victory would likely only be short-lived. The machine learning capabilities available to search engines and the regularity of their updates mean any exploitable weakness you may find are going to be patched quickly.
In short: relying on exploiting search engine’s algorithms with clever tricks is not an effective long term SEO - or broader marketing – strategy.
Moz’s Rand Fishkin explains it well but to summarise: if you chase the algorithms religiously, you are likely to lose sight of the larger goal of appealing to your human audience. When the search engines (who are ultimately trying to predict what humans will like) change their metrics to decide this - and, rest assured, they will! - the chances are you will come up short and all that effort will be for nought.
Let me illustrate with an example.
Imagine that you have the means to infer to a virtually undeniable level (which is very difficult, far beyond the capability of a correlation study) that Facebook 'likes' are directly used in Google’s algorithm. Naturally, you develop a strategy to capitalise on this and base a large portion of your efforts on getting 'likes'. Focusing aggressively on 'likes', less user-friendly tactics start to seep into your strategy - things such as hiding content until they like the page or producing mediocre content just for likes; users are put off and not really engaging but you have the likes and that’s all that matters, algorithmically. Basically, you start teaching to the test.
All goes well until but Google suddenly decides – by virtue of its ongoing evaluations (or, indeed just arbitrarily) - that Facebook 'likes' are no longer so valuable. Your strategy is exposed and your rankings drop to what they once were. Worse still, because your approach has been neglecting your true audience, your rankings continue to drop. You’ve been burned.
And that’s before we even consider the fact that Google’s analysis may already have them implementing an algorithmic update before you’ve finished your study and rolled out your strategy. The size, speed and sophistication of search engine evaluations are almost certainly going to outstrip your capacity to exploit loopholes.
Understand the limits of correlations
The vast majority of people will be able to tell you that correlation does not imply causation. There is nothing particularly special about correlation studies in this sense - truth be told, no statistical model truly implies causation. There will always be a level of uncertainty over what is actually causing something to happen.
There is an underlying problem with mean spearman correlations though. Mainly in what way(s) they can be interoperated with.
The figures given in mean spearman studies are not the correlation of one sample for each variable. They are the summary of a set of correlations. When one sees for instance, the number 0.22 associated with the variable “Total number of links to page”, that 0.22 is not a correlation. It is the attempt of the person conducting the study to summarise his or her, possibly very large, collection of correlations.
Separating the correlations in this manner initially is perfectly valid. After all, it is not sensible to attempt a numerical comparison between a web page being in the fifth position for “fancy dress costumes” and another one being third for “cheap hotels in London”.
The temptation is to try and summarize all these correlations by a single, more digestible number. And, on the face of it, averaging them may seem valid. To understand why this can be problematic, we must first understand why we may choose to average our data in the first place.
What exactly is an average?
Normally, when we average a set of numbers we are estimating an unknown parameter. We do this because averaging has been shown to have a lot of favourable properties in these contexts; mainly that the number it produces is often an unbiased maximum likelihood estimator of our parameter and easy to calculate.
To put it simply: when we average a set of data we are actually just guessing some unknown number and given the right circumstances, this average can be mathematically shown to be best possible guess based on our data.
For example suppose you have a fair die. The “true” probability, P, of rolling a five is assumed to be a sixth. Imagine, however, that you don’t know how to work out this probability – you’d need to set an experiment to estimate your (now unknown) parameter P. You roll the die 100 times and record the numbers of fives (N). Based on this experiment what would your estimate of your parameter, P, be?
Anyone with a grasp of statistics will know, intuitively, blurt out “N/100” but can you explain why?
Clue: the answer is not as simple as “because it’s the average” - it actually involves a lot of sophisticated maths to explain that for estimating P from your 100 rolls your best option is to go with N/100.
Averaging in correlation studies
But things become even more clouded when we wish to average correlation coefficients. What exactly is even being estimated? What is the equivalent of our P in the above example? And how does estimating this parameter help us in answering our underling questions?
Unfortunately a lot of people use them to interoperate with what they want them to give information on or suggest. Some of the most commonly claimed questions they are claimed to help answer are
- What variables are more prevalent in higher ranking pages?
- Can any of the variables be used to predict page positions?
- Which variables improve my chances of ranking highly?
- What do search engines think is popular with users?
And the problem here is there is a fundamental gap of logic between knowing the mean of a collection of correlations and being closer to answer these questions.
For more details on some of the more technical points of correlation studies and an alternative method specifically for the question “which variables improve my chances of ranking highly?”, I’ve gone into more detail in this piece where I explore whether logistic regression may be better suited to answer the question.
So what CAN I take from correlation studies?
We have to accept that it can be more challenging that we’d imagine to use correlation studies to make concrete declarations.
However, that does not mean that a correlation study cannot teach us things for digital marketing. It just means we have to live with a level of uncertainty and, as with many things in life, base our decisions on broader context and experience.
So how can digital marketers make the most of these studies?
- 1. Recognise that search engine positions are a means to an end
They are not the ultimate goal. Your audience is.
- 2. Avoid over evaluating the numbers
Unless you are ready to start working with the raw data there really is no use in trying to fit a narrative to number of Google “+1”s having a mean spearman of 0.34 and Facebook 'Likes' having one of 0.27. The appropriate interpretations are not fully understood so be cautious of anyone claiming otherwise.
- 3. Use them in combination with other sources
Correlation studies are likely to be more suited as a jumping off point or a means of establishing where to focus more specific enquiries. No matter how impressive the sample size or aesthetically pleasing the graphics, it’s unlikely you’ll find an overwhelming case for a theory – as such, using them as stand-alone evidence is not advisable.
- 4. Be confident enough to ignore them
Any statistical study or model will work on a set of assumptions and a level of inaccuracy. They are not a guarantee for individual success especially if the assumptions are, on full reflection, not met.
Two common assumptions of correlation studies are namely that searches are location blind and that the webpages have already attained a minimum position for a search term (e.g. top 50). This means, for example, a small accountancy firm in Manchester ranking around 200th on Google for the query “Manchester accountants” should probably not base decisions purely on these correlation studies as they are for now not ranked highly enough. It is also unlikely that that their target audience is turning off local searches.
Summary of Correlation Studies
The sand under the feet of digital marketers is ever-shifting – as such, it’s impractical to expect studies to match science journals for longevity. The processes take too long and internet trends can come and go much more quickly than other traditional marketing mediums such as television or radio. As such, we have to compromise a level of certainty to be able to take advantage of these trends in time.
Even with that caveat however, I’d always be sceptical with correlation studies in their current form. That is not to say correlation studies are wrong or not useful. Only that their usefulness is, so far (statistical safety valve!), formally unknown and you should ultimately be aware of this before overcommitting to the numbers they produce. Always remember to view these studies in the larger context and above all else, always put your human audience first.
Thanks to Kristian Petterson for sharing their advice and opinions in this post. Kristian is the Marketing Manager at Search Marketing Group, a UK-based agency focused on maximising reach and conversion potential through bespoke search marketing and active analysis of best practice. You can follow SMG on Twitter or connect via LinkedIn.